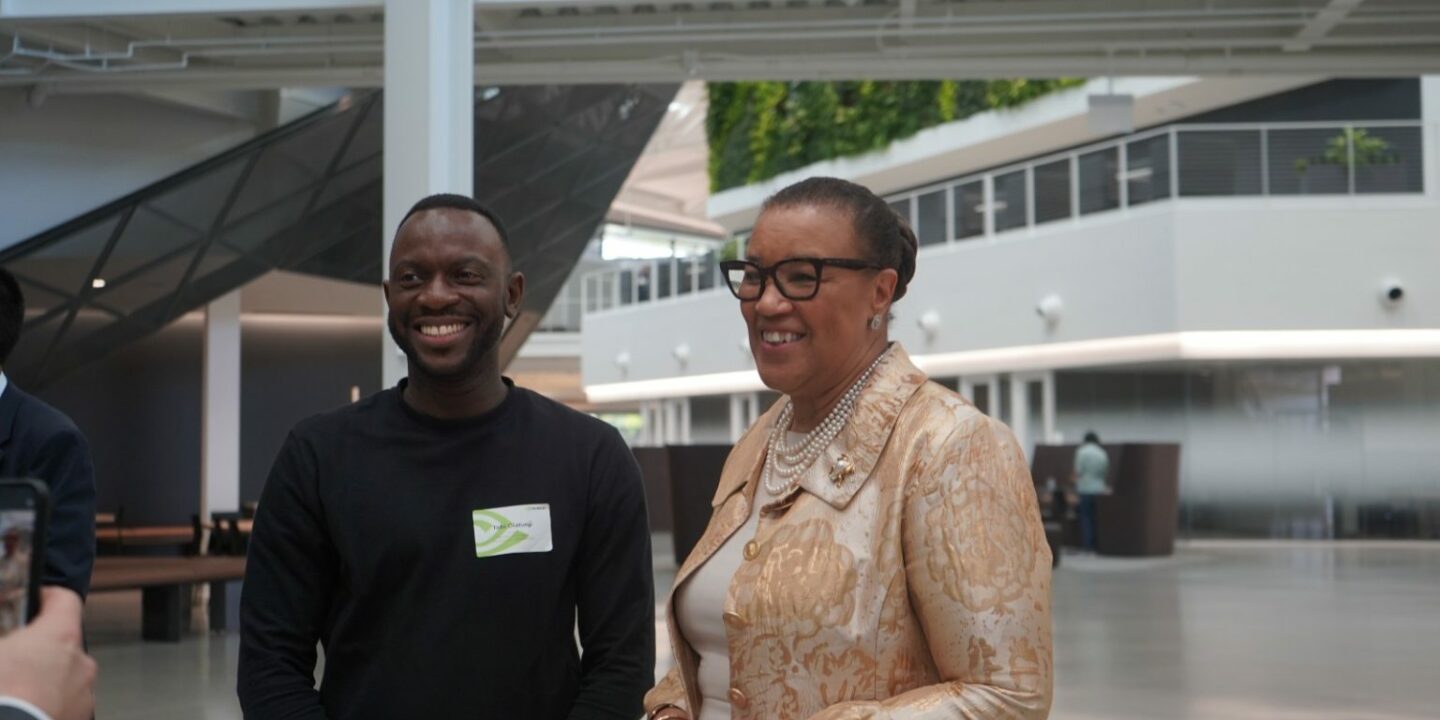
Source: Abraham Augustine/Techcabal
Africa does not have enough healthcare personnel. So healthcare workers are expected to take care of more patients per doctor than in more developed economies. Paperwork takes up a significant portion of time on the job. Healthcare workers need to take patients’ medical histories, fill forms and update these records with time. Modern hospitals are digitising how they take these records but a computer and some software do not necessarily make the job easier. Sometimes, it takes up too much time and doctors go back to writing their notes on paper.
One Nigerian-trained doctor and AI specialist believes that artificial intelligence can help African hospitals digitise medical records faster and save time for doctors.
Nigeria, Africa’s most populated country, needs 363,000 doctors to achieve universal healthcare coverage. But Nigeria only has 24,000 licensed medical doctors. In 2017 when Nigeria needed 237,000 doctors to meet the World Health Organization’s (WHO) recommended doctor-to-patient ratio, the country had even more— 35,000 doctors. The number of qualified medical doctors has fallen by 31.4% despite the population growing by 2.46% on average between 2017 and 2021.
As a result, too few doctors see far too many patients. Doctors in Nigeria frequently complain of burnout and being “overused and underpaid”. The story is the same across much of Africa. With approximately 3.6 million health workers in the 47 countries, the WHO estimates that Africa has a ratio of 1.55 health workers (including physicians, nurses and midwives) per 1,000 people. The recommended threshold is 4.45 healthcare workers for every 1,000 patients. By 2018, only four countries (Mauritius, Namibia, Seychelles and South Africa) had surpassed the WHO health worker-to-population ratio.
Digitising healthcare
In the early 1960s, the Mayo Clinic in Rochester in the US state of Minnesota was one of the first major healthcare centres to adopt an electronic health record (EHR) system. It was expensive and basic and could only be used to manage patient appointments and billing. Since then, electronic health record software has become much more sophisticated, allowing for detailed information about the patient’s health to be collected and processed.
EHRs are not widely used in African healthcare despite their much-talked-about benefits. Installing EHR systems and training healthcare workers to use them is expensive. It is also often blamed for the lack of adoption of EHR tools by hospitals. But there are subtler reasons. Tobi Olatunji, a University of Ibadan-trained doctor turned computer scientist, says even in hospitals where EHR systems are installed they are not always used because doctors find them cumbersome and time-consuming. Doctors may be computer literate, but “when you put a keyboard in front of people, then that’s a whole different problem that you are creating.”
So Olatunji co-founded Intron Health, a startup that uses automatic speech recognition (ASR) technology to transcribe doctors’ notes while they speak. But Intron Health did not start out with speech-to-text software. Intron Health, which was founded in 2019, offered a regular EHR software solution to help hospitals digitise their processes.
In 2020, as COVID-19 spread globally and healthcare workers worried that Africa’s frail healthcare system could easily be overwhelmed, Intron Health piloted its first software at a Nigerian hospital. “It was a busy hospital and they were all excited. They had electricity; we had installed a wireless network for them. Everything was great,” Olatunji recalls. “[But] the day we launched, the doctor spent 40 minutes just to type the notes for the first patient that came.” Seeing the next patient took 50 minutes and by this time patients in the waiting room were getting visibly frustrated. If a doctor cannot see patients because they need to use clunky computer software to create or update medical records, waiting patients will be tempted to seek help elsewhere. The hospitals using Intron Health’s early software asked them if they could simplify it by replacing text boxes with checkboxes. But that was a crude solution and it meant you had to predict every possible medical situation to create a robust enough checkbox system. That was impossible.
During his days as a medical student at University College Hospital (UCH) Ibadan, Olatunji had faced a similar frustration during an anatomy course where a lecturer struggled to explain how babies pass through the birth canal with only textbook pictures and hand gestures. He felt a video lesson would be better and students could repeat the lesson as much as they needed to. Somehow he was able to convince the university to build a rudimentary learning management system with hosted animated video lessons, with funding from the United States National Institutes of Health (NIH) and the World Bank. From then on, Olatunji’s path began to diverge from medical practice. His university asked him to help train staff from other universities, and when he graduated, he was employed by the university to build technology tools, including telesurgery tools, a patient navigation app and clinical simulation software.
From UCH Ibadan, Olatunji made his way to the United States where he got a master’s degree in medical informatics from the University of San Francisco and another in computer science at Georgia Tech. He was employed by Enlitic, a San Francisco Bay Area company, as a machine learning scientist and researcher to help build natural language processing (NLP) and natural language understanding (NLU) AI models to help translate English text to other languages. After leaving Enlitic, he joined the Health AI team at Amazon Web Services as a machine learning scientist. On the side, he was already building software to digitise hospital records in Africa, which became Intron Health. But his first rodeo ran into a snag. Doctors in Africa who saw too many patients could not type fast enough for a digital medical record system to make an EHR installation worthwhile.
Keyboard to microphones
Drawing inspiration from speech-to-text software that Olatunji’s wife, also a doctor, used, Intron Health tested popular speech-to-text platforms and found the obvious. They all failed to properly transcribe African-accented pronunciations and names. “I tried them out myself and we realised that those things were not built for our accents. If doctors used them, it would waste too much of their time,” Olatunji explains.
But he had worked on these types of problems so the Intron team decided to build a proprietary speech recognition platform and embed it in EHR platforms to make taking notes easier and free up valuable time for the doctors.

This was 2021 and the Intron community threw itself into sourcing for African-accented audio datasets. Intron has now built up more than 11 million voice samples from 7,000 speakers in 13 countries and more than 200 unique accents. It allows them to build automatic speech recognition software that can translate accented speech with more fidelity than Google Assistant, Siri or Alexa, for example.
When Intron onboards hospitals to its platform, it asks healthcare workers to read a short 74-word paragraph. “Most people take up to 55 seconds to dictate this and speech speed is pretty constant,” Olatunji explains. But the doctors could take as much as 5 minutes to type out the same paragraph as they pick at their keyboards, one letter at a time. With speech recognition technology that understands African accents, doctors can take notes faster and save time. According to Olatunji several hospitals in Nigeria, Kenya, Ghana and South Africa are now using Intron’s speech-to-text software.
Nakunta Muwasu is a medical doctor at Meridian Health Group in Nairobi, one of the hospitals using Intron Health’s software. “It makes taking short notes faster,” Muwasu tells TechCabal. But for longer records, she prefers to type on her keyboard.
Intron has produced several papers under review which it intends to present at computer science academic conferences to showcase their research and product. In one of the papers co-authored by Tobi Olatunji, Tejumade Afonja and five other computer scientists, the authors show how their African-accent model outperforms the more popular speech recognition software models.

Intron is one of the African startups using artificial intelligence to deliver digital transformation. Earlier this year, InstaDeep a Tunisian-born enterprise AI company, which was founded in 2015 and raised $107 million from investors, was acquired by BioNTech for $680 million. Intron, by contrast, has only raised a family-and-friends round of $250,000 since its inception in 2019 to date. Despite this, it has managed to build and maintain the expensive infrastructure requirements for running an AI company—partly thanks to a partnership with GPU chipmaker, Nvidia.
As part of the same partnership, the company is currently running a $5,000 award hackathon, inviting programmers to build better models using a small percentage of its training dataset. Afri-Speech-200, the developer challenge was created in partnership with DSN, Masakhane and Zindi to advance diversity in artificial intelligence. The lack of diversity and the potential for bias is one of the problems AI practitioners and technology regulators have had to grapple with. Intron’s ASR technology may prove to be a valuable tool for African healthcare workers, but it also highlights the work that needs to be done to ensure that AI systems are not completely blind to contexts outside developed countries where training data is abundant.
Source: Abraham Augustine/Techcabal